First of all, let me introduce the 5C approach to unpacking a science question. This was developed in collaboration with a few other Science senior teachers in Singapore, namely Dr Bernard Ng (ST Chem, Edgefield Sec), Mr Shah Dhaval Prahhaker (ST Physics, Hai Sing Catholic High), Mrs Kim Loh-Teo (ST Physics, Guangyang Sec) and Ms Jacqueline Ng (ST Biology, Nan Hua High School). This question-answering approach is meant to serve as a scaffold for students to draw connections between new contexts and prior knowledge in order to answer higher order thinking questions.
While exploring customised GPTs, I attempted to integrate the AI’s capabilities with the 5C thinking process in order to flesh out the 5 components of the process. I also uploaded the syllabus document for the O level sciences for retrieval augmented generation so that the responses can make specific mentions of the topic titles and concepts’ key ideas. The prompts given to the GPT was also quite detailed. Here’s how it works:
1. Context: Simplification and Summary
When a question is presented, either in text or image form, the AI first simplifies the question. This involves summarising the main ideas or concepts present in the question. For example, if the question is about the principles of thermodynamics, the AI might summarize this as “a problem relating to heat transfer and energy conservation.”
2. Chapter or Topic Identification
The AI, using its understanding of the syllabus and educational material, will then identify the most likely chapter or topic that the question is based on. This helps in narrowing down the scope of the content and focusing on relevant material.
3. Concepts: Keyword Integration
Next, the AI lists out the key concepts or keywords relevant to the topic, as found in the syllabus document. For instance, in a question about cellular respiration, keywords like “mitochondria,” “ATP,” and “glycolysis” might be identified.
4. Connect Concepts to Context
The AI will then demonstrate how these keywords and concepts can be applied to answer the question. This involves showing the connection between theoretical knowledge and practical application. For example, linking the concept of “ATP” to the energy requirements in cellular processes.
5. Construct Solution: Guided Problem Solving
Rather than providing a direct answer, the AI guides the user in constructing their solution. This might involve suggesting steps to solve the problem, such as breaking down the question into smaller parts, applying formulas, or suggesting methods for analysis.
As an example, the image of a question on Dynamics was given. The AI was able to correctly simplify the context, identify the relevant topic and concepts and suggest an approach to solving the problem.
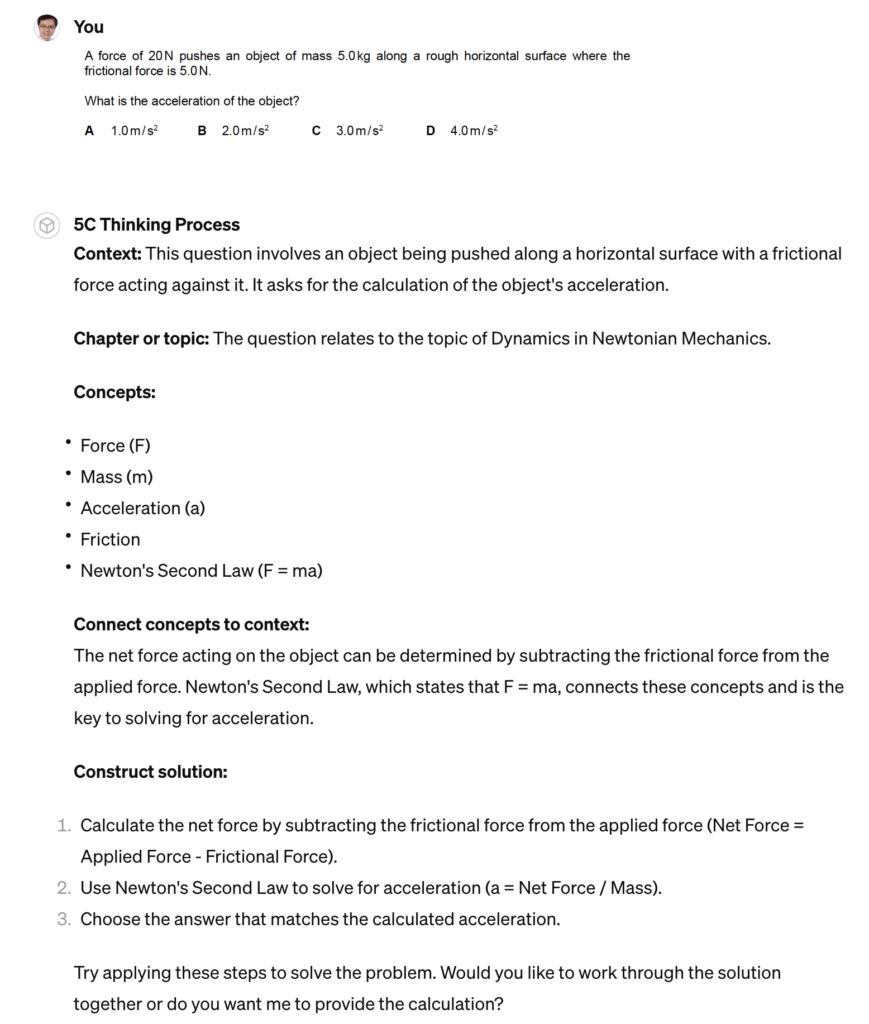
Providing Solutions Only Upon Request
The AI will only provide a direct solution if explicitly prompted by the user. In such cases, if the solution involves mathematical problems, it will be presented using LaTeX generated math working to ensure clarity and precision. After providing the solution, the AI encourages the user to try a different problem for practice and consolidation of learning.
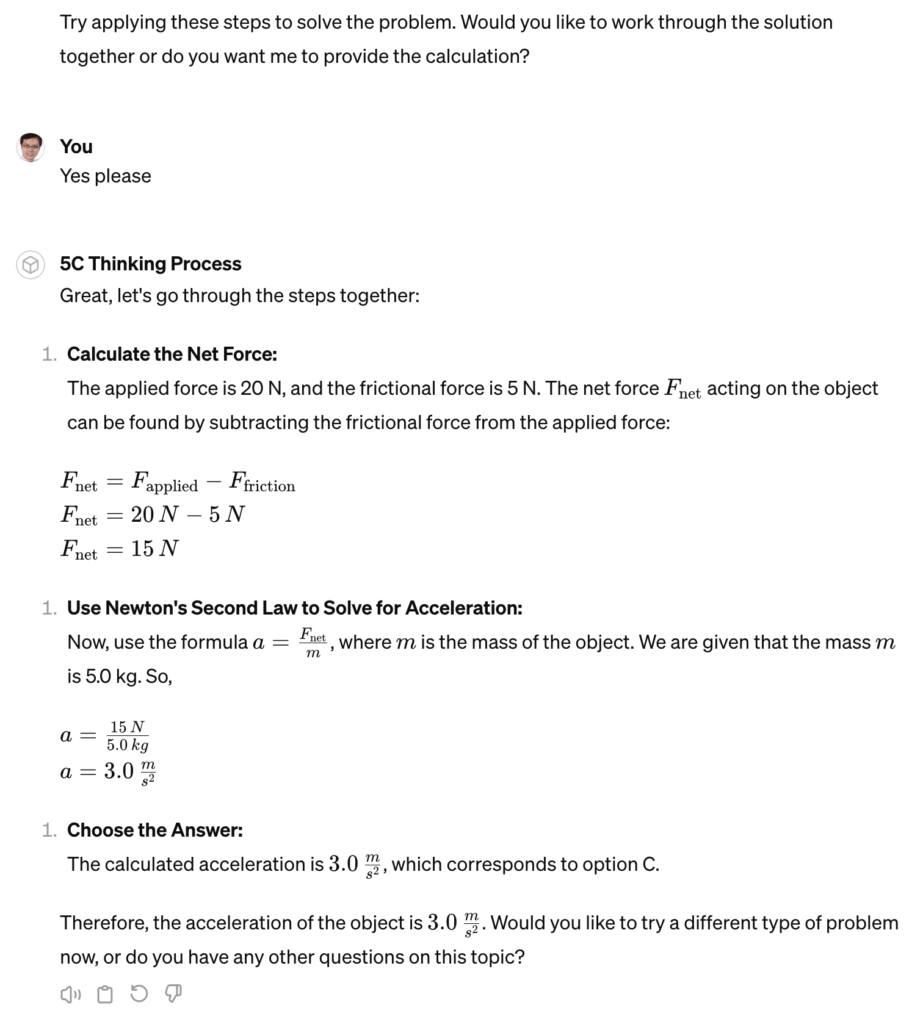
Generation of Similar Questions
Finally, if the user desires, the AI can generate similar questions. This allows for further practice and helps in reinforcing the concepts learned. The limitation for this last task is that it cannot produce similar images if the original question involves graphs or diagrams. Therefore, it will only work for word problems such as the following.
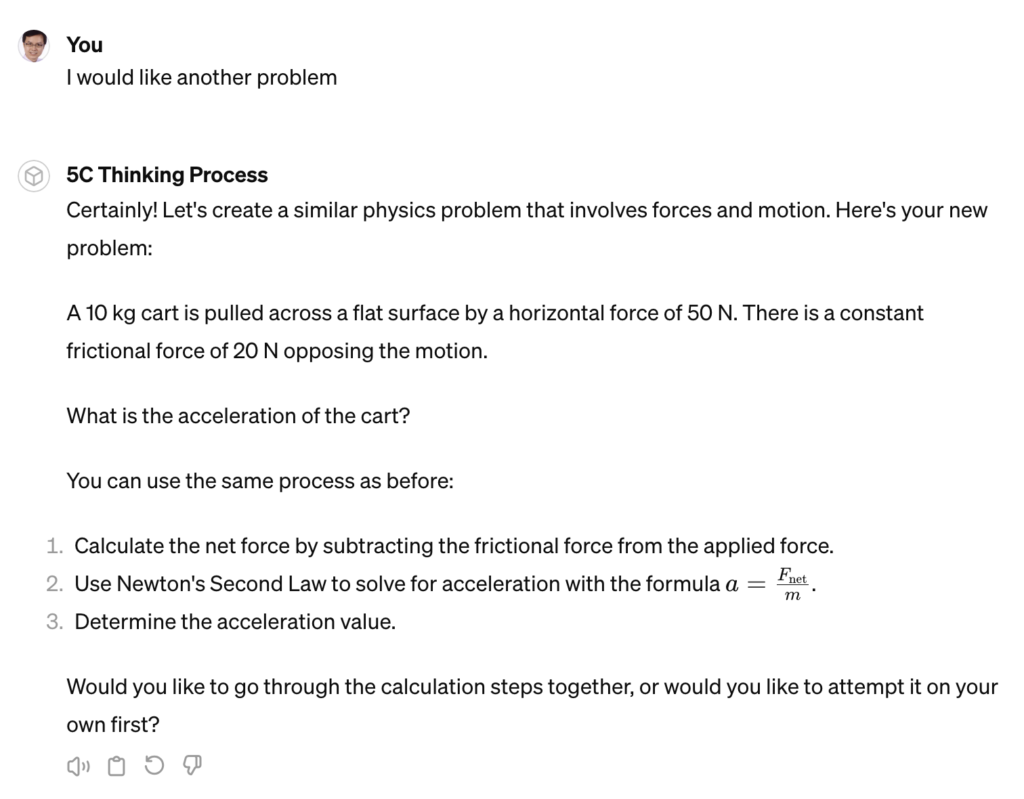
This approach not only makes the learning process interactive and engaging but also ensures that the understanding of concepts is deep and thorough. It empowers students to be active learners, enhancing their problem-solving skills and conceptual understanding.
Higher Level Problems
I then “trained” the AI with the ‘A’ level Physics syllabus and tested it out with questions of the same standard.
It was able to answer a question on how stationary waves is formed, but over-delivered unnecessarily by elaborating on concepts like wavelength being twice the distance between adjacent nodes when the question did not ask for it.
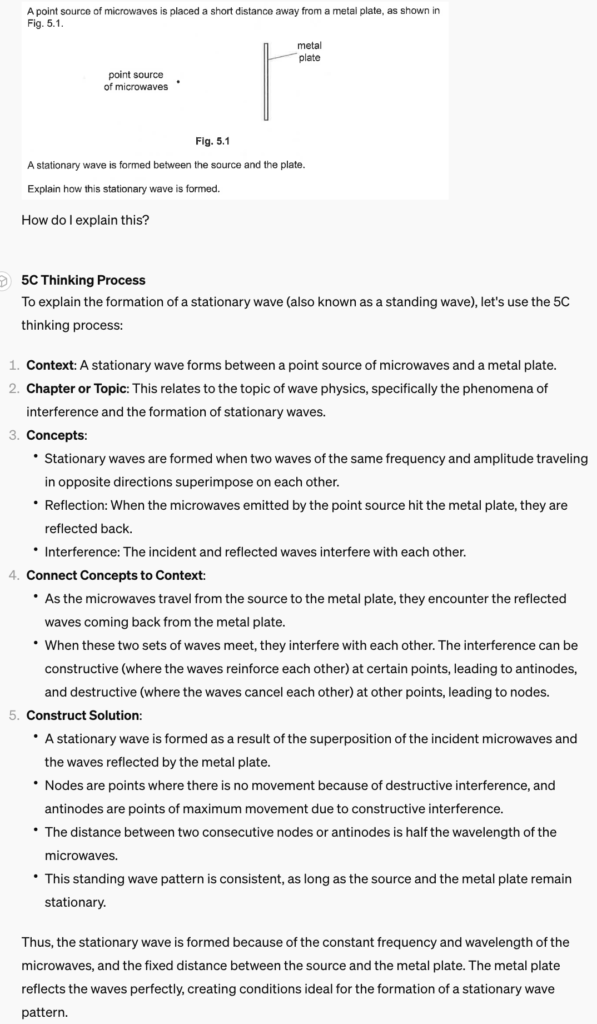
The next question on the path of a moving object entering a uniform field and moving in a parabolic path requires the AI to learn that from the diagram. It did so impressively.
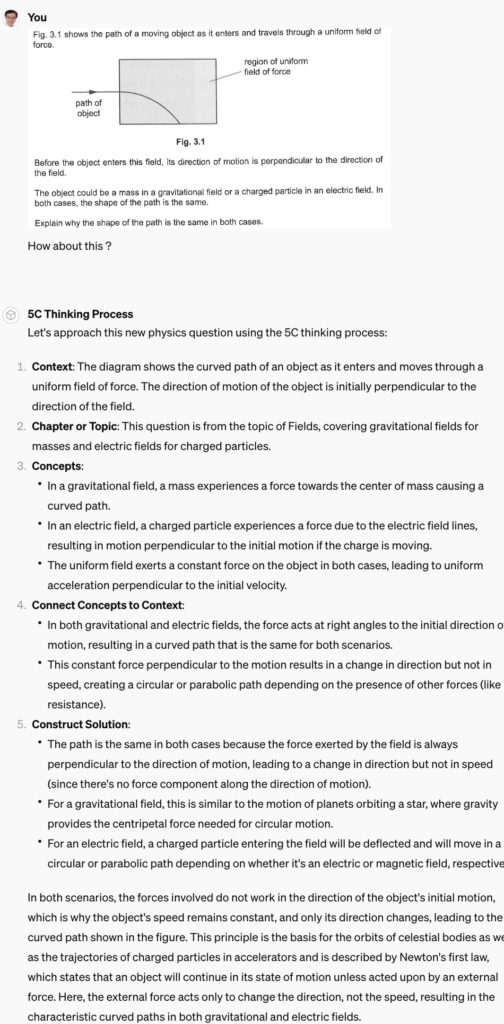
The next attempt did not go so well. It involves an object travelling down a rough slope in a two-part question. This required the AI to infer that in the stem of the question, the object is held in place with friction opposing the parallel component of weight. The label for the rough slope could have hinted at it but the AI failed to see that. Therefore, it was not able to answer part (ii) of the question accurately.
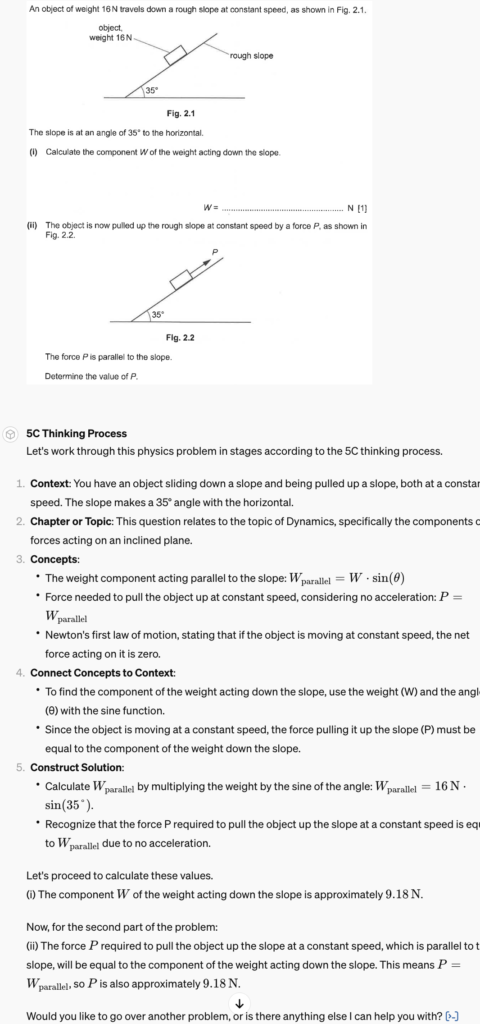
Despite the occasional gaffes, customized GPTs hold significant potential in aiding students to unpack science questions, making the learning experience more interactive and engaging. These AI models, tailored for educational purposes, can offer customised scaffolds provided by the teacher and assistance to the students, personalised according to learning preference and pace. Other advantages include:
- Students might also be less fearful of making mistakes in front of an AI.
- AI’s ability to offer immediate feedback so students can more quickly understand and rectify their errors.
- The conversations can be limited to discussions about the content so that students do not abuse the system.
Deployment
These AI models with the ability to process questions in the form of images can be a game-changer. One platform for teachers to build and deploy the AI is using the paid version of Poe.com allows teachers to customise a GPT-3.5 bot and deploy it to students. To deploy a GPT-4 bot, students will have to pay as well.
If one is not willing to pay, Poe.com’s Gemini-Pro GPT can do something similar for a limited number of responses but might be less accurate. Do try it out if you are an educator who is curious about how education can be radically transformed with AI.